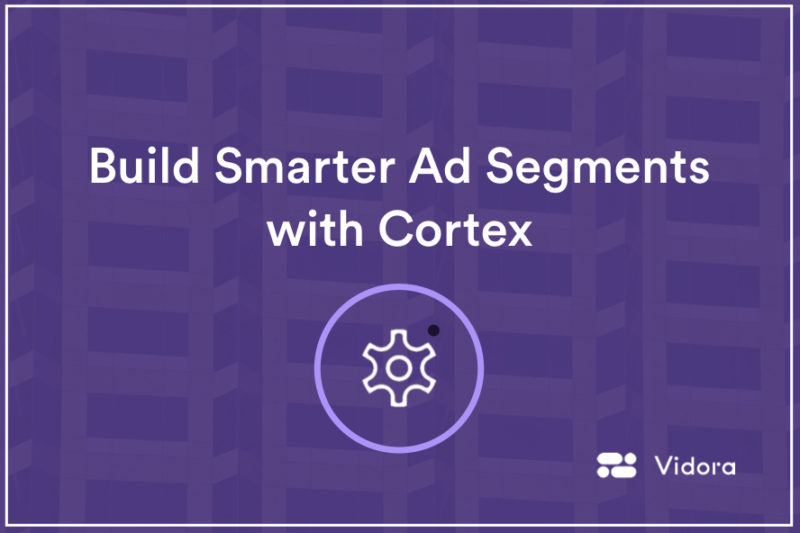
Launching a successful advertising campaign is a balancing act. Advertisers want to reach as many people as possible, but also need to ensure precise targeting so that their ad will not fall on deaf ears.
In recent years, Look Alike modeling has been billed by ad-tech providers as the ideal solution to this dilemma. In practice, however, Look Alike models can produce poorly targeted segments that we at Vidora believe should be used only as a last resort. Instead, Vidora Cortex offers various other Machine Learning approaches (including Classification and Uplift) that are much better suited to provide high-performing ad segments.
What are Look Alike segments?
As the name implies, the goal of a Look Alike model is to find an audience that “looks” similar to a known set of users. There are two core components of any basic Look Alike segment –
- Seed Set: Which set of users should the Look Alike audience be based off of?
- Segment Size: How large should the audience be? A larger audience means a broader reach, but less overall similarity to your seed set.
For example, an advertiser might use a set of 1,000 known homeowners (the seed set) to build an audience of 50,000 Look Alikes (the segment size) who are similar to those homeowners.
Limitations of Look Alike Models
The simplicity of Look Alike modeling has led to widespread adoption by ad-tech platforms such as DMPs, Ad Exchanges, and social networks. But what’s often not highlighted by these providers is that Look Alike modeling brings significant limitations which often hinder ad performance.
To illustrate these challenges, let’s consider an example: Say a commercial bank has just launched a new business loan product, and would like to advertise toward a segment of 10k small business owners (SBOs) on our site. Here’s the data we have at our disposal –
- There are 1m active users on the site
- Of these 1m users, 100k have registered a profile, and specified various pieces of demographic information (including job title) during the registration process
- Of these 100k users, 2k indicated that they were small business owners (SBOs)
Limitation #1: Lack of Behavioral Data
The first limitation we’ll run into is that most ad-tech platforms make Look Alike predictions based exclusively on user attributes (e.g. age, location, gender identity, etc.). This information is only readily available for our registered users, so the model won’t even consider 90% of the total population when constructing a Look Alike segment.
Limitation #2: Lack of Negative Labels
Look Alike models are typically built via an approach that focuses on users in the seed set , without any consideration for those not in the seed set. This means that Look Alikes are prone to incorrectly finding a trait that is shared by the seed set, yet is irrelevant to the targeting we’d like to do.
Returning to the example above, this problem shows up in that small business ownership is not the only trait that our seed set users share; they’re also all registered users. Registered users may behave very differently from unregistered ones, so our model will likely latch onto this discrepancy and produce a Look Alike audience composed entirely of registered users, regardless of whether they are small business owners. The model performance metrics might indicate high quality, but the campaign will perform poorly in the real world.
Other (Better) Alternatives
At Vidora, our experience with Fortune 500 advertisers has taught us that other ML-based approaches typically lead to superior campaign results. Look Alike segments do have their place in the ad world — in fact, Vidora Cortex offers Look Alike modeling which blends user attributes with your unique first party behavioral data in order to build segments with broader reach and a higher level of accuracy. Still, we believe that Look Alike modeling should be used only when other, better alternatives are unavailable.
Among these alternatives are Classification, Conversion Predictions, and Uplift — all of which can be built in minutes using Cortex’s no-code interface.
Alternative #1: Classification
Classification is a common type of ML which seeks to divide two groups of data. By learning from info about both who is and isn’t in the seed set, Classification usually leads to better ad segments than Look Alikes.
Recall that in our example above, Look Alike modeling was insufficient because the seed set shared a trait that we weren’t looking to identify in other users. Instead, say we use Cortex to build a Classification model which differentiates the seed set from a separate negative set –
- Positive set: registered users with job title equal to “small business owners”
- Negative set: registered users with job title not equal to “small business owners”
Both sets contain registered users, so the model can’t simply rely on the behavior of registered vs. unregistered users to divide the two groups. Instead, we’ve forced the model to differentiate between users based on the relevant factor: are they likely to be a small business owner?
Create a Classification model in Cortex is as easy as uploading a file
Alternative #2: Conversion Predictions
If the goal of your advertisement is to drive a particular user action, conversion predictions may be an even better choice. These predictions enable advertisers to target users who are most likely to take a particular action, such as engaging with the ad.
To illustrate, let’s say that our example bank now wants to optimize its campaign for clicks in order to gather sales leads. A segment of likely SBOs identified via Classification may be a decent proxy for those who might click, but it’s not perfect. Some SBOs simply aren’t in the market for a loan. Other non-SBOs may be considering starting a business, and would want to learn more about the bank’s loan product. Using conversion predictions to find which users are mostly likely to click can help determine the right targeting strategy for these users.
Use Cortex to predict any user action over any window of time without any coding
Alternative #3: Uplift Predictions
If the bank’s end goal is to influence customer behavior downstream in the conversion funnel, Uplift predictions are the best choice. An Uplift model predicts how each customer will respond to a particular intervention — say, a targeted ad.
Why is this useful? Even among users likely to click, the effect of the ad (in terms of likelihood to take out a loan) might vary. For some, the ad may persuade them to transact. For others, it might have no effect. Others still may come away with a worse impression of the bank, and will be less likely to take out a loan than they were before. Uplift can identify these cohorts to make sure that you target only those users for whom the ad will drive incremental conversions.
Cortex is the only platform which offers no-code Uplift modeling for ad tech teams
Want to learn more?
The well heralded demise of 3rd party cookies creates additional imperatives for businesses to fully leverage the power of their 1st party data. Most businesses are now familiar with Look Alike modeling, but in practice other ML techniques often yield better results. Vidora Cortex makes these techniques accessible to any ad-tech team, enabling your organization to offer premium ad segments without the challenges of dealing with big data.
For more information, reach out to info@vidora.com or read our recent article on ad segments featured in Towards Data Science.