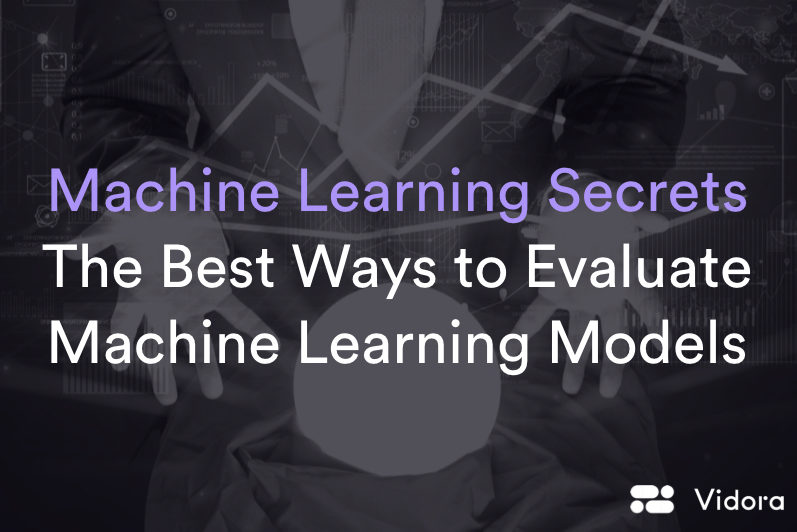
Machine learning is increasingly the cornerstone of success for numerous marketing and product teams. The ability to harness predictive insights is invaluable to optimize and automate customer experiences. Some common use cases include: segmentation and targeting, customer churn, and predicting future events. Given that machine learning is increasingly being used to drive key business outcomes, a natural question follows : how do we evaluate the performance of the machine learning models? In this video by Vidora’s Shawn Azman, we walk through some of the challenges and solutions to understanding the performance of your machine learning models.
The Challenge
How do business teams know that the predictions made by their machine learning models are accurate? For example, consider if you build a machine learning model to predict which of your users will purchase shoes in the next 7 days in order to market a new shoe line to those customers. How can you gain confidence that these predictions are accurate and that they should be used in a production setting?
The Solution
There are actually several ways to measure the performance of your machine learning model ranging from creating a hold out set of data, to running the model over various time periods. Each evaluation method has its own pros and cons which Shawn steps through using Cortex in the video below.
Gain Confidence in ML Predictions
Want to learn more about leveraging your machine learning predictions for your marketing and product teams? Email us at partners@vidora.com or fill out our contact form!
Contact
Contact us using the form below to learn more about Vidora. We’re excited to hear from you!