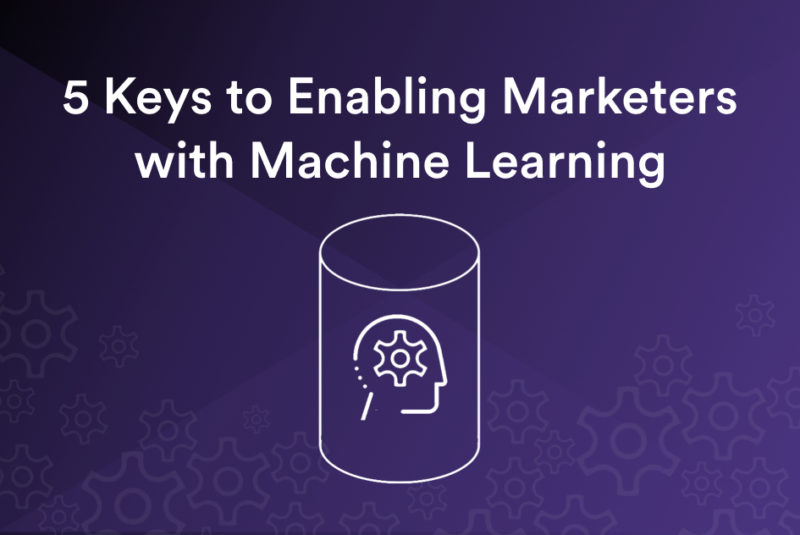
Machine learning offers a powerful set of tools for marketers to optimize and automate engagement with customers. However, many organizations struggle to directly empower their business teams, including marketers, with machine learning due to technical hurdles. This is a problem for businesses: the marketing teams have the best understanding of the customer challenges they are looking to solve with machine learning and ideally would have machine learning tools directly at their own disposal. New no-code machine learning platforms are finally opening up the possibilities for businesses to directly empower marketers with machine learning.
Here we review 5 of the biggest technical challenges marketers face when deploying machine learning.
Getting Data In : Machine Learning for Marketers Marketing Challenge #1
Typically the first challenge confronting a marketer is how to provide the machine learning platform access to data. Often this data is stored in data lakes or data warehouses but not formatted in a way that a machine learning platform can easily leverage. Transforming data from it’s raw format within the storage locations to a format which a machine learning model can easily leverage is often termed “data wrangling”. In general, for any machine learning problem, data wrangling is the most time consuming step of the machine learning process. However, new no-code tools, focused on particular verticals like marketing, are able to automate much of the data ingest and data wrangling for marketers.
Getting Data Out : Machine Learning for Marketers Challenge #2
Machine learning models generate predictions about the future. Examples include –
- Will a user engage on a particular marketing channel?
- Will a user churn in the next 60 days
- What’s the likelihood a user will be up-sold to a new service?
These predictions, in order to be actionable, should be sent to various parts of the business – ideally with only a few clicks.
Destinations include –
- Data warehouses and data lakes
- Marketing automation platforms
- DMP and CDPs
- Etc
Often these destinations require the data to be in a particular format in order to be ingested and, in addition, require particular login credentials in order to access the destination. For a machine learning platform to be accessible to marketers it’s important that the platform enable a variety of marketing integrations and handle the formatting and authentication steps needed.
Turn-key integrations to data warehouses and marketing platforms.
Specifying Business Goals : Machine Learning for Marketers Challenge #3
Every business is unique and the marketers at each business have the best understanding of what the goals are for their business. The machine learning platform should be flexible enough to enable a marketer to specify a variety of goals and iterate on those goals.
Consider, for instance, a typical machine learning goal for a marketer which is predicting user churn. There are various ways to define churn and each marketer is in the best position to define churn at their company. For instance should churn be predicting on a 5-day, 30-day, 90-day time-frame. Alternately the marketer might want to define churn as user inactivity over a 30 day period. And so forth.
Ideally the no-code platform would provide an easy interface for a marketer to specify and optimize for various business goals.
Screenshot from Vidora Cortex of a marketer specifying a model goal.
Understanding Model Performance : Machine Learning for Marketers Challenge #4
How well did your machine learning model perform? Evaluating model performance can be a tricky proposition. There are a variety of, often obscure, machine learning metrics which can be used to evaluate a model. These metrics often are used in conjunction with an ongoing performance on live data to evaluate the quality of a model. A machine learning platform should present the performance of a model in a clear and easily understandable manner. Here is blog post which reviews some of the challenges associated with model evaluation.
Continuously Evaluation and Learning : Machine Learning for Marketers Challenge #5
The final challenge is that most models are not static but should be continuously evaluating and learning from the latest data. Consider, for instance, predicting user churn and using churn scores to help define a marketing campaign. The ideal scenario is for the churn model to be continuously predicting which users are at-risk rather than only evaluating risk once. Building this type of ongoing evaluation can be difficult when paired with the challenges outlined above.
Surmounting Machine Learning Challenges and Empowering Marketers
The challenges described above are formidable and often impose significant barriers to marketers adopting machine learning technology. However, new platforms and technologies are making machine learning accessible to a broader spectrum of business users. Once marketers are using machine learning technology on a daily basis the result will be a dramatic increase in productivity through automation and new revenue opportunities through optimization.