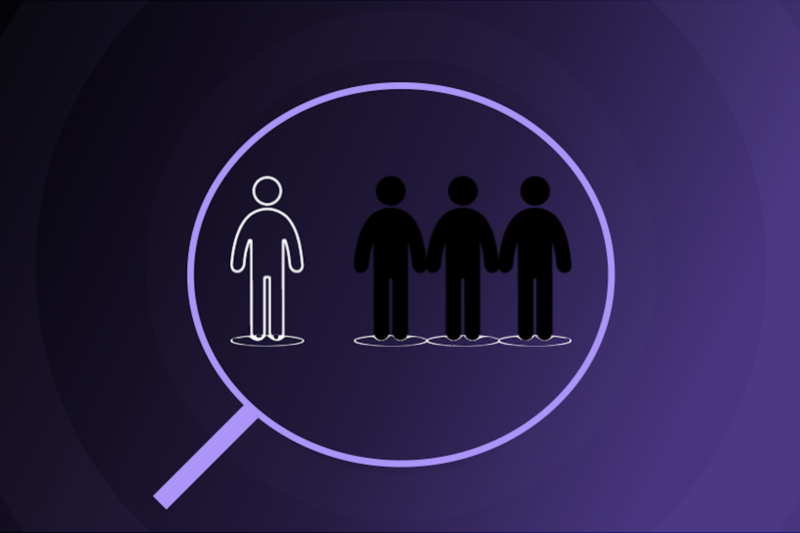
Uplift Modeling is an important machine learning technique which every marketer and product manager should be aware of. In many cases, marketers might want to consider using uplift modeling over more traditional machine learning techniques like propensity modeling.
In this blog post we will provide a brief overview of uplift modeling and then provide several examples of how uplift modeling is used by marketers to optimize the metrics they care most about.
Note that Vidora provides uplift modeling techniques as part of our no-code machine learning Cortex product. You can learn more about how to leverage uplift modeling in Cortex here. Also note that uplift modeling, when contrasted with propensity modeling, is often referred to as “prescriptive modeling”, while propensity modeling is referred to as “predictive modeling”.
Uplift Modeling Overview
The easy way to think about uplift modeling in a marketing context is that it predicts the impact of an action on a user. In this case we can think of “impact” as the result of taking that action on the user, resulting in a “uplift” in their conversion probability or conversely negatively affecting their probability. Uplift models are notably different from more standard machine learning models like propensity models which measure the likelihood of an action happening (i.e. the likelihood of churn, the likelihood of purchase, etc). Uplift modeling goes beyond propensity modeling by predicting how acting on users will change the users’ likelihood of churn, likelihood of purchasing, etc.
Uplift Modeling in Practice
In the following section we list out some common marketing problems where machine learning can be applied and how they can be viewed as uplift models.
Example 1 – Uplift Modeling for Churn Reduction
Churn reduction is a key metric for nearly every business. Naturally marketers are interested in understanding which techniques will reduce their customer churn. Marketers typically have a variety of techniques at their disposal ranging from email campaigns, to offers/discounts, to having a customer representative directly call the customer. The natural question is, which marketing technique will reduce a customer’s churn probability the most?
Uplift modeling provides a natural solution. With uplift modeling a marketer can calculate the impact of each marketing technique on a particular customer’s churn probability. For instance, Customer A may have a biggest decrease in churn when a customer representative directly calls them while Customer B might have the biggest decrease in churn probability when they receive an loyalty offer. Uplift modeling can tell you.
As a side note, how does propensity modeling fit into the mix of uplift modeling techniques? Churn propensity modeling will tell you how likely each customer will be to churn, but it won’t easily tell you which marketing technique to use to reduce churn the most.
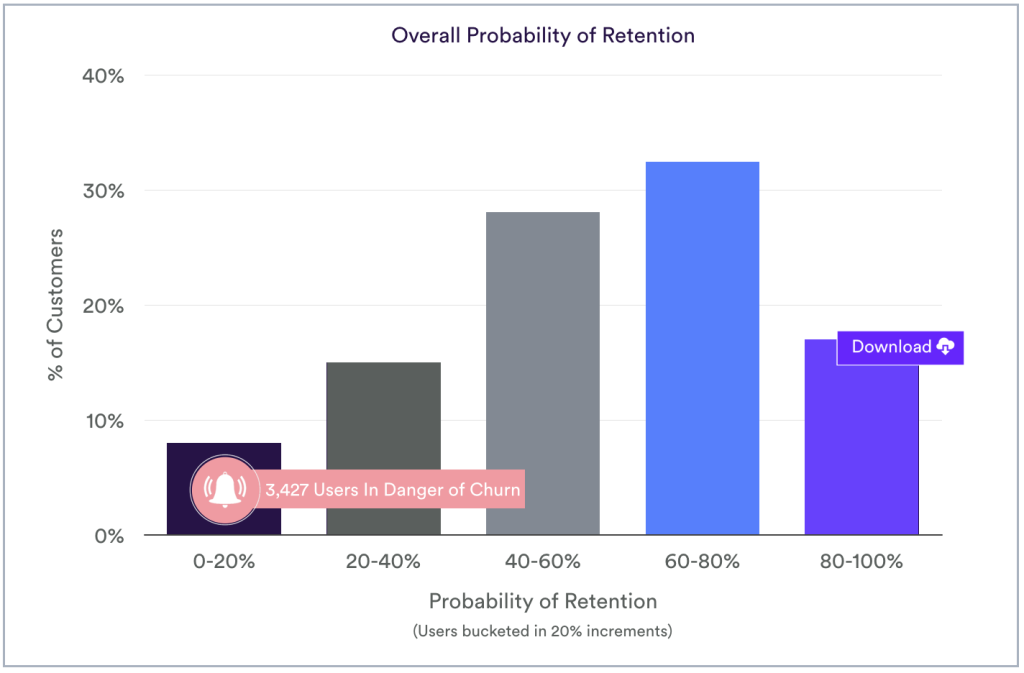
Example 2 – Uplift Modeling for Increasing Purchases
Financial services and commerce companies are often looking to provide additional value-add services and products to their customers. Marketers naturally ask the question of which of the numerous marketing techniques available to them will increase the likelihood of a user converting the most?
Uplift modeling can play a key role here as well. Uplifting modeling can tell a marketer whether, for instance, an email, a phone call, or direct mail will increase the likelihood of a customer converging the most.
Propensity modeling, on the other hand, will tell a marketer the likelihood of a customer purchasing a new service or product. Propensity modeling will tell you that Customer A is more likely to purchase a product than Customer B but uplift modeling will tell you how to increase the likelihood of Customer A purchasing the new service the most.
Example 3 – Picking the Right Offer to Send
A common marketing technique is to send customers (or prospects) offers to incentive them to purchase a new service. Marketers naturally would like to send users offers which will incentivize users to purchase while at the same time not discounting the service too aggressively. As an example, should a marketer send offer A offering a $10 discount, or offer B offering a $20 discount to a particular customer?
Propensity modeling can help a marketer understand who is most likely to be receptive to a discount, but uplift modeling shines by helping a marketer understand how much each offer will impact a user’s decision to purchase. If offer A and B both increase a users likelihood of purchasing the service by the same amount, then the marketer can save $10 by sending the customer offer A. That’s $10 saved!
Uplift Modeling and Cortex
Uplift modeling is an exciting technique to explore for marketers which can result in significant ROI in the right applications. At Vidora we are committed to providing a machine learning platform (Cortex) accessible to marketers and product managers which enables them to explore the benefits of techniques like uplift modeling without any coding or machine learning experience. If you are interested in learning more, please email us at partners@vidora.com.