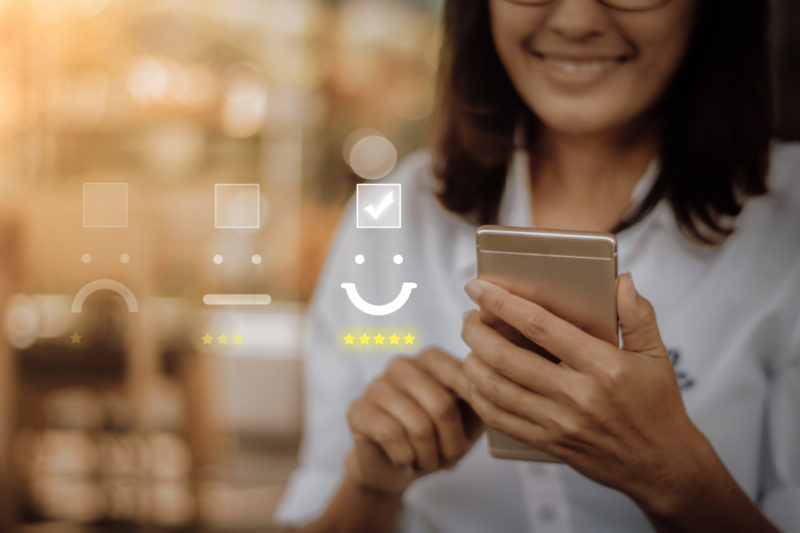
Customer retention is one of the most important aspects of any business. In the past posts we have described how to build customer retention machine learning pipelines from event data. In this post we’ll focus on understanding customer retention.
Building a Customer Retention Pipeline
Cortex makes it easy to build a customer retention pipeline based off of event data (here’s a post on how to build a predictive pipeline). Typically, one can build a retention pipeline by predicting whether a customer will have any interaction in the next 30, 60, or 90 days (see the image below for how to specify a retention pipeline in Cortex).
Build a Future Events pipeline in Cortex to predict each user’s probability of remaining active within the next 30 days
Understanding the Key Drivers of a Customer Retention Pipeline
Feature Importance conveys which features are most important for understanding whether a particular customer is retained. For instance activity on an online site might be a good indicator of customer retention. Alternately whether a customer downloads the mobile app might be another important indicator. The indicators for your business will typically be unique. You can easily download Feature importance within the Cortex interface for a particular pipeline.
Feature Importance for a customer retention pipeline
Downloading Features – Understanding Customer Retention
Next it may be useful to understand why a particular user was retained (Feature Importance will tell you which features are most important across all customers and not why a particular customer is retained).
To download features for all customers simply download them here.
Feature Downloads option
At this point you can look at each individual customer to see the values of important features for that particular customer.
Sample image of a CSV with customer ids and various features in the columns
For instance if activity in the last 30 days ends up being an important feature once can see the value of this particular feature for a particular at risk user over the last 30 days. By combining Feature Importance with downloaded features one can develop a comprehensive understanding of what your most and least loyal customers are doing.
Are you looking to understand your customer’s behavior to improve your business? Reach out at info@vidora.com to start a conversation!