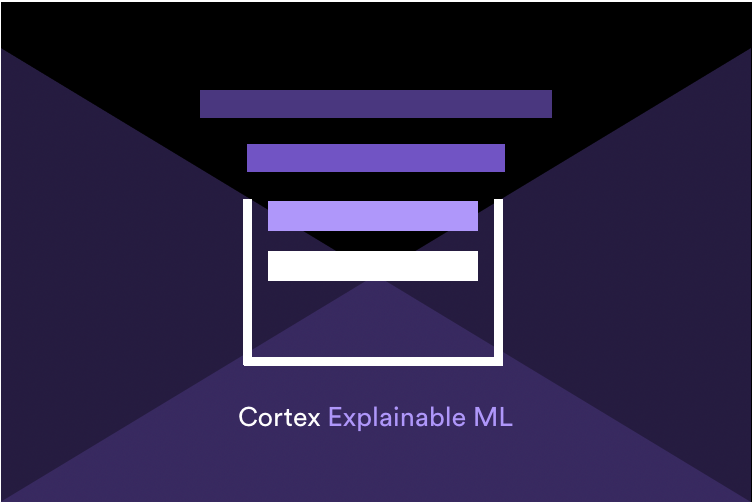
Machine learning. Everybody is either doing it or trying to get their data organized so they can start. The list of use cases that ML can be applied to is ever-growing. Today, businesses are picking them off one by one. But while the machines are getting smarter and helping us intelligently automate more and more tasks, we don’t always have the proper visibility into how they made their predictions. The truth is, for many, ML is a black box. We throw data in and get predictions out. However, it’s hard to understand how the process came to the predictive conclusions that it did. So how can we become a part of the feedback loop? How can this be a two-way conversation with the machines? The answer is Explainable ML.
Today, efforts are being made within the industry to open the ML black box. This means we provide more transparency in the machine learning process, and give us insight into why the results turned out the way they did. One name for it is Explainable ML. In Vidora’s case, customers need more than just predictions. They also need to understand what it was in their data that resulted in those predictions. What were the big influencers? How might my predictions change if I made other adjustments to my business? How can I explain it in layman’s terms to the rest of the organization?
For example, businesses that want to predict churn need to know more than just who is likely to churn. They need to know the reasons why. Therefore, you can build strategies to alleviate it further and get the team behind the cause. If you’re a video subscription company, maybe churn is directly tied to how many videos users watch in a specific category. What if you were told that increasing plays for that category by 1% could have a 2% reduction of churn? That would be invaluable information. Or maybe you’re a real-estate business and you learn that a 1% increase in form submissions would increase a customer’s likelihood to buy a property by 5%.
Machine learning is a great tool for making predictions. However, Explainable ML takes it one step further by informing us about how it’s using the data and how we can turn those insights into new company-wide strategies.
See What’s Happening on the Inside
Needless to say, Explainable ML is a cornerstone of Vidora’s Cortex platform. Our goal is to make ML accessible to all teams, regardless of their technical chops, and Explainable ML is a big part of what makes this possible. The platform becomes easier to use for everybody the more that we expose the inner workings of the ML process and explain the decisions it’s making. With Explainable ML, everybody can become an expert with the data being used to build ML models, they don’t have to spend time analyzing complex charts to get answers, and they can drive new initiatives by sharing the learnings with the team. Though not exhaustive, the list below provides a couple of examples of how Cortex and Explainable ML can help you open the black box:
- Feature Importances: View your model’s features ranked by importance to understand which ones are most predictive of your company’s objectives.
- Feature Impact: See estimates for how influencing each feature can positively impact your model’s goals.
Methods to explain ML are constantly evolving, and we’re looking forward to working with all of you to further define what types of Explainable ML features would be helpful within your organization as you deploy machine learning solutions. Have any ideas? Drop us a line at info@vidora.com. We’d love to hear what will help you and your team think better and think faster, just like the machines.