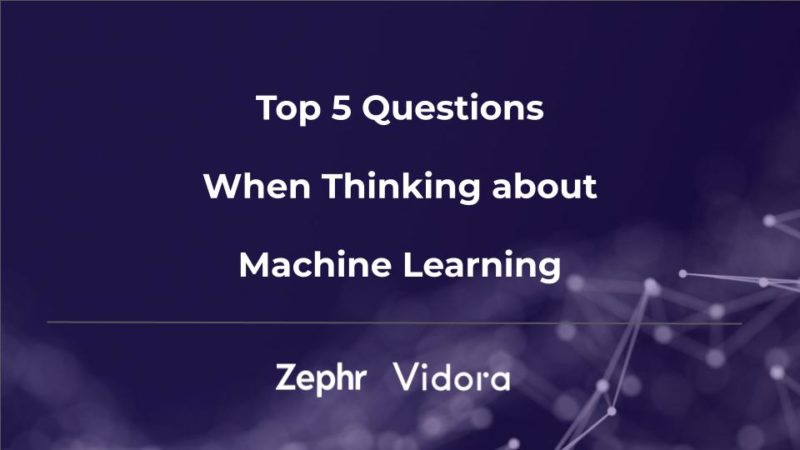
Businesses are making big bets to collect and manage vast amounts of their data. As the amount of data increases, companies are considering how Machine Learning fits into their data strategy. At Vidora, we work every day with companies to operationalize Machine Learning into their existing data workflows. Some customers we work with have previous experience turning existing data into predictions, while many others are just getting started with Machine Learning for the first time.
Below, we consider some of the common questions from customers evaluating Machine Learning, and provide learnings we’ve had along the way to help you decide if Machine Learning is right for your business.
Question 1: Do I Really Need Machine Learning?
Given all the hype around Machine Learning, it’s common to find a healthy dose of skepticism as to whether Machine Learning can really move the needle for their business. To help answer this, we find it’s best to focus on a specific example to show some of the strengths of Machine Learning, and we’ll use an example common to almost all businesses: Customer Segmentation.
When segmenting users and customers, the idea is often to create a group of similar users to learn more about them or to take some action with them. And very often, the use of this is forward looking: “segment the most likely customers to churn in order to give them an incentive to stay with the business longer”. And it’s questions like these where Machine Learning can often provide additional benefits, for two main reasons:
- ML Uses All Available Data: Teams usually focus on a few key data points for segmentation that are clearly related to the question at hand. However, by limiting our analysis to only a subset of data points, we are eliminating the idea that any of the other data can provide additional value to our analysis. ML can use all data present and find the most important data points automatically, finding connections in data we could not.
- ML Continuously Learns and Adapts: Manual segmentation usually finds learning about the past and assumes those will apply to the future. But what if customer behaviors change? Being able to adapt to changing environments is a strength of ML that is constantly evolving and learning.
In short, ML enables continual learning from the past to predict the future. As the market and customers change, ML changes with it by using all data available to find the most predictive behavioral patterns. If you have large sets of data and an ever adapting market, Machine Learning could be right for you.
Question 2: What Data is Best for Machine Learning?
Given how broad the field of AI/ML is, the correct answer to this question could be ‘anything and everything’. But given Vidora’s expertise in creating Machine Learning for consumer business, we’ll stick with what is the best data for creating customer predictions!
Before we talk about the actual data sets, it’s important to talk about data hygiene. There are two areas we focus on which helps set companies up for success:
- Consolidated Data Sets: given customers can interact with a business across multiple platforms, having one data set representative of all customer activity is crucial.
- Common User IDs: in order to stitch together data from multiple platforms, a common User ID ensures all activity is attributed to the same customer.
As for the data that produces the best customer predictions, having access to raw customer behavioral data is best. This should include:
- Conversion Events: These are the primary events in your customer funnel, e.g. subscribe, register, purchase, churn, etc. Other ancillary events and behaviors can also be tracked to give a fuller picture of the customer’s preferences, but key conversion events are necessities.
- Customer Attributes: While events say what customers are doing, attributes help inform who customers are. This information can help Machine Learning find attributes that are most predictive of one outcome or another.
- Item/Content Catalogues: Since many consumer businesses have products or content that customers are interacting with, knowing which items are available and which a specific customer has interacted with can provide the foundation for user recommendations.
Question 3: How Does Machine Learning Work?
A common connection people make is that Machine Learning is equivalent to a model. And while the model is the primary aspect of Machine Learning that is responsible for making the prediction, there is much more that goes into having a product Machine Learning deployment than just training a model. We often divide this into 5 steps:
- Data Wrangling: Gathering and consolidation of data internally. It’s important to have a full view of the data, e.g. across all platforms, and consistency across the data, e.g. unified User IDs.
- Feature Engineering: Choosing what data to use for the model is often the hardest part of Machine Learning. This requires transforming the raw data into features required by the model.
- Model Selection: This step is about finding the best configuration of a model which produces the most accurate result.
- Prediction Generation: The final step of automation is generation predictions on a per-user basis based on the model selected from the previous step.
- Operationalize: After validating the model and determining the predictions are accurate, the final step is to operationalize the model to be used in the real world. This allows the model to continually ingest new data, at production scales, and generate new high-fidelity predictions on an ongoing basis.
Question 4: How Do I Know a Model is Good?
This is a question that needs to be answered for every new Machine Learning model that is built. Because of this, we work with customers on a three step validation process for every new prediction:
- Step 1 – Test Prediction Accuracy on Historic Data: Since models use historic data to create predictions, this first step when building a new model is to test how accurate it is at predicting events that happened in the past. For example: if you predict who will churn, have the model make the prediction for a time in the past and use known churn data to determine the accuracy.
- Step 2 – Track Prediction Accuracy in the Future: Once predictions are shown to be accurate in the past, the next step is to track their accuracy in the future. This helps show that the learnings the model gained from the past are actually predictive of behaviors in the future.
- Step 3 – Test Predictions in Production: Having accurate predictions is only half the battle, as now the team has to put those predictions to use. For customer predictions, this can often be accomplished in an A/B Test to see if making decisions based on predictions is better than decisions made manually.
Assuming the Machine Learning model proves accurate at each step, the final step in the process is to operationalize and deploy the predictions. This includes the automation and retraining of the model as well as making the predictions available for continuous use to the business.
Question 5: How Do I Best Take Action on Predictions?
The next step is to put these insights into action, through hyper-personalized user journeys. Using predicted behavior insights, commercial decision makers can build informed conversion strategies that call on enriched data to segment and target different segments of users effectively.
Often this requires the capabilities of another solution. In the digital media and publishing space, for example, our partners Zephr help leading businesses manage every aspect of the subscriber lifecycle, from acquisition to retention, using deeply personalized paywall strategies and user experiences. Customization at the granular level means that the on-site experience of every reader can be tailored to their needs, preferences and predicted behaviors, from the content they are shown to the packages they are offered.
The ability to deploy and experiment commercial strategies based on visitor data and predictive analytics leads to a more aligned value proposition between the news provider and the reader, resulting in higher conversion rates, longer subscriber lifetimes and reduced customer churn.
What to Learn More?
If you want to learn more about how to utilize Machine Learning for your business needs, please reach out to us at info@vidora.com!
We have also partnered up with Zephr to make it as simple as possible to operationalize your Machine Learning Predictions live on your site. They have recently written an article outlining the best practices to think about when operationalizing your predictions, so we encourage you to give that a look as well!