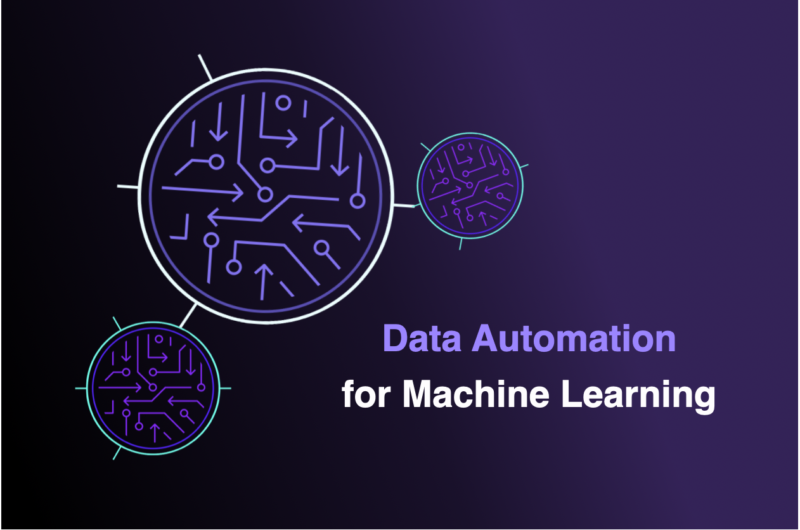
We are living in an era of unprecedented change. Beyond the pernicious advent of black swan events, technology’s relentless pace of innovation is creating an increasingly dynamic market. This dynamism generates an ever-changing deluge of data. Businesses looking to stay competitive in the market require techniques and technology to intelligently process and transform data quickly. The ability for a business to to drive automation and optimization will depend on its adoption of these technologies.
Machine Learning Enables Rapid Business Adaptation
Machine learning and related AI technologies hold tremendous promise to enable businesses in intelligently leveraging their first part data for automation and optimization. Continuous machine learning is a type of machine learning capable of adapting to changing market dynamics. As a result, continuous learning is uniquely positioned to be a nexus technology for competitive businesses which are driving decisions through rapidly changing market data.
Data Science Automation for Machine Learning
Some of the main challenges organizations face when adopting machine learning include both (a) the large investments of development time needed to transform large amounts of raw data into formats amenable data for machine learning, and (b) garnering the organizational resources required to undertake those data transformations. These challenges can make machine learning both ineffective and prohibitive cost-wise for many companies. From a performance standpoint, the tedious time investments needed to transform data often result in the algorithms using stale information. This staleness is a consequence of the algorithms not taking into account the latest dynamic market conditions. In addition, it is increasingly challenging for companies to hire qualified data scientists. This hiring challenge results in many projects not being undertaken or being dramatically under-resourced.
The general data manipulation tasks associated with machine learning are often bucketed under the umbrella of data science. To be sure, the data science tasks required for machine learning algorithms are a unique subset of the broader data science space. Data science as a discipline subsumes diverse areas ranging from visualizing business metrics to calculating monthly revenue totals. However, data science for machine learning is a very unique flavor of data science. In particular, the data science steps for machine learning can be generally summarized within the framework of a “Machine Learning Pipeline” (see this article for an introduction to Machine Learning Pipelines). At Vidora, we are focused on automating the data science tasks associated with Machine Learning Pipelines. Cortex, our product, provides the platform for businesses to pursue Machine Learning Pipelines. By automating various parts of the Machine Learning Pipeline, Cortex empowers businesses to quickly deploy machine learning technology across a variety of use cases. It also allows data scientists to focus their skills on problems not amenable to automation.
View of Cortex and the various types of Machine Learning Pipelines it supports
Data Science Automation – Which Businesses Stand to Gain?
Most businesses will benefit from increased data science automation. However, specific verticals stand to benefit more than others. In general, verticals which generate a large amount of high quality data are most likely to realize strong benefits from automation and optimization associated with continuous machine learning. Vidora works with companies across Financial Services, Real Estate, Enterprise Media, and Commerce. All of these companies share the common attributes of collecting large amounts of high quality 1st party data.
Cortex automates data science tasks associated with building Machine Learning Pipelines. Above is a view of a Cortex and a Machine Learning Pipeline which continuously adapts to large-scale data.
Data Science Automation – What Does the Future Hold?
The space of data science automation, as it specifically applies to Machine Learning Pipelines, is still nascent and relatively unexplored. In the coming years, business necessity will drive an increasing amount of innovation in this space. To be sure, in the short and medium term there will be limitations to what can be automated. Specific problems sets will be more susceptible to automation. Whereas some problems, in particular those with unique business dependencies, will be more resistant to automation. That being said, over time, we expect the power of data science automation tools to increase and provide increasing benefits to businesses. Businesses which adopt these technologies will enable their teams to participate and thrive in an increasingly dynamic market.
Learn More
Interested in learning more? Check out a demo by reaching out to us at info@vidora.com.