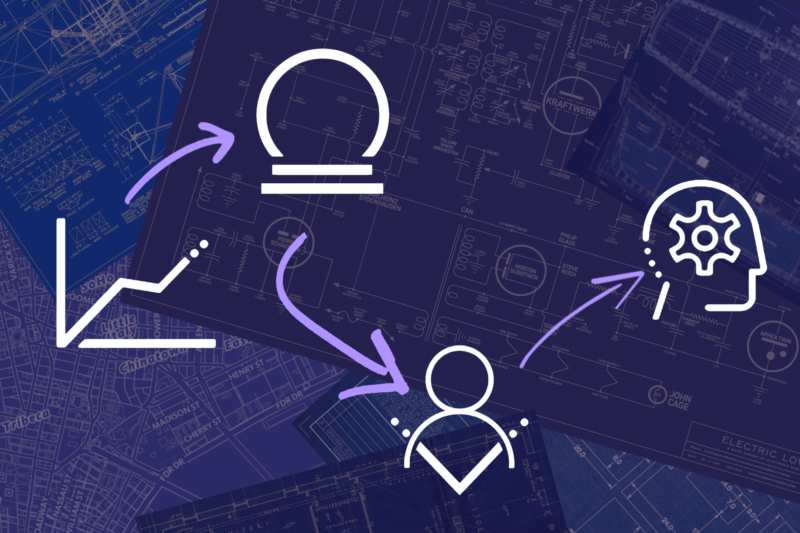
Over the past few years, Vidora has been working with some of the world’s largest businesses to develop and implement a Machine Learning (ML) system for each that gives anyone access to this complex technology. Today, Artificial Intelligence (AI) and ML solutions already sit at the heart many of the world’s leading businesses. And today, this field continues to be one of the most innovative in the world.
Over the course of engaging with various businesses, we have noticed certain patterns in adopting Machine Learning Technology. Typically, we can group business into one of the following four stages:
Data Consolidation
Today, most businesses recognize the essential value of collecting and storing data on their users. They also recognize how important it is to ensure that data is in one easily accessible central location.
Effective data consolidation can be a daunting and expensive undertaking. This is especially so for businesses that have relied on legacy systems to store their data. However, the main challenge we see in businesses is that data often lies in disparate silos within the organization. You need engineering investments to drive that data into a single central location.
It is important to note that data consolidation is a process that can take years. Additionally, this remains an ongoing process. We typically encourage businesses to begin using Machine Learning technology early on. We do so in order to fully demonstrate its value to the organization. By doing so, businesses put themselves in a position to further increase the amount of consolidated data they have access to with ML technology.
Prediction
With systems in place to consolidate data, the inevitable priority becomes doing useful things with it. For many businesses, visualizing that data represents the logical next step, and tools like Tableau and Looker are common products used in the market. However, businesses know that data is only really powerful when you can be use it to (a) predict future outcomes and (b) automate and optimize processes.
Prediction is particularly appealing to organizations. This is because it allows them to set longer term strategies, rather than take a reactionary approach to their data. ML technology is an effective way to make predictions on vital questions. Some of those questions include predicting CLV, churn, who should receive offers, what content to recommend, and so on. But implementing ML well is not trivial for most organizations. Building a system to generate predictions means businesses will often run into issues with data not being clean, difficulties with feature engineering, and many more (here is a Vidora blog post which highlights these difficulties and how to address them).
Automation
When an organization begins to have confidence in the predictive results they see from ML, the next stage involves tying those predictions more closely to feedback loops without human interaction. We call this the “Automation” phase of ML. By creating these feedback loops, an ML system is able to dynamically learn from the data independent of human intervention.
Creating a feedback loop for data and predictions provides an adaptive system that continuously learns and improves. This is not only in terms of predicting certain outcomes, but also in allowing businesses to automate and optimize the way they act on those predictions. An ML system that can for example predict which users are most likely to make a transaction would then be able to automate and personalize email sales campaigns so that those users are shown the products they are likely to buy. To successfully enable automation requires confidence from the organization that the system will continue to work well without human supervision.
Strategic AI
The final stage in the ML evolution of a business is to enable AI and ML technology for every person and every aspect of the organization. This even includes those with no data science or engineering background. You need a mature understanding of the data to get to this stage of implementing Strategic AI. As well as this, the implications it carries for the cross-functional groups interested in leveraging it. It also requires the implementation of advanced techniques such as No Code Automated Machine Learning (No-Code AutoML).
AutoML goes far beyond simply selecting which machine learning model is best for a particular prediction or automation objective. Instead, AutoML automates most of the steps required to implement machine learning at scale. This includes steps such as data cleaning, ingestion and engineering, feature engineering, model generation and selection, and many more.
While AutoML is a relatively new field of exploration in AI, it is one where we are already seeing rapid progress. And with products such as Vidora’s Cortex at the forefront of machine learning’s latest progression, leading companies are already surging ahead to automate and optimize their entire business with the power of ML.