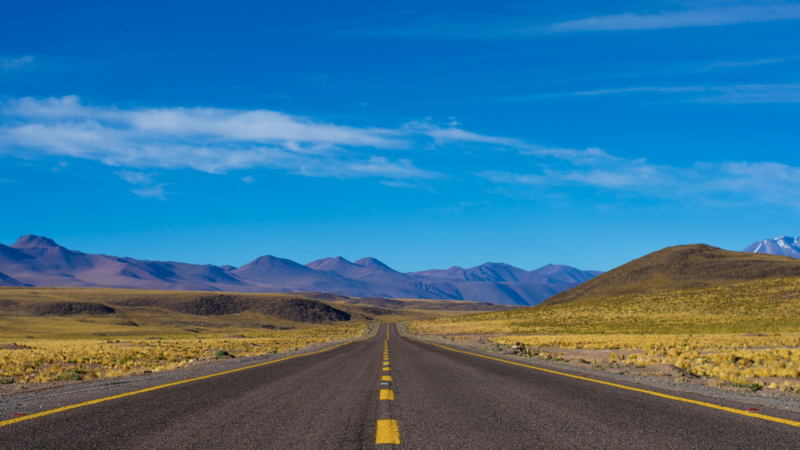
Dynamic decisioning enables businesses to build user journeys which are tailored uniquely to individual users. Machine learning can be a powerful ally to any dynamic decisioning experience. Machine learning allows the decision points to learn and adapt to user behaviors over time, thereby ensuring a level of optimality in terms of conversions. If you want to ensure that you are maximizing conversions, machine learning is almost always the way to go.
Common dynamic decisioning use cases include optimizing paywall conversions and increasing conversion from anonymous to paid users.
But getting machine learning powered dynamic decisioning right can be a tricky proposition! In fact, often dynamic decisioning is not implemented correctly meaning the business is missing out on valuable conversions.
Two machine learning areas we’ve found to be important to ensure the highest number of conversions are Uplift Modeling and Real-Time Inference. The benefit, when these techniques are used correctly in conjunction with dynamic decisioning, is high assurance that the maximum number of users are being converted.
Uplift Modeling vs. Predictive Modeling
Uplift Modeling enables a business to optimize for the impact of an intervention on a user. In dynamic decisioning parlance an intervention would include whether to show a hard paywall to a user or whether to pop up a registration dialogue to a user. Uplift Modeling will tell you, for each user, how much that intervention will increase a user’s chance of converting.
Uplift modeling is the optimal method to use for most dynamic decisioning experiences. I.e., typically a business wants to ask “If I show a paywall at this moment, will that increase the likelihood of a user converting and by how much will that likelihood increase?”
However, many businesses leverage Predictive Modeling in lieu of Uplift Modeling. Predictive Modeling will provide a prediction on a user’s likelihood to convert in general – independent of any intervention. Predictive Modeling doesn’t actually convey information on whether to show a paywall at a particular moment or not! Uplift Modeling, on the other hand, will tell you whether showing a hard paywall now will result in an increase in a user’s likelihood of converting and how likely that user will be to convert after they experience the change in user experience (such as seeing a paywall).
Here’s a good primer on the benefits of Uplift Modeling.
Real-Time Inference
For dynamic user journeys in particular leveraging in session (real-time) data is a critical component for maximizing conversions. Whether a user will convert is often heavily dependent on real-time features like a user’s activity within a session, the time of day, or the device a user is on. Ideally both real-time features and past behaviors would be used to make dynamic decisions on a user journey.
Here is some more info on real-time inference.
(Above) Cortex provides an interface and platform for quickly building and deploying dynamic decisioning experiences. This pipeline is designed to maximize user conversions using Uplift Modeling and Real-Time inference for paywalls.
Putting It Together – Uplift Modeling and Real-Time Inference
Combining more sophisticated machine learning techniques like Uplift Modeling with Real-Time Inference enables a business to build user journeys and conversion funnels which act optimally on each user and ultimately maximize conversions. It’s definitely exciting times in the world of machine learning for user journey optimization.
If you are thinking about dynamic decisioning, Uplift Modeling, or Real-Time Inference let’s talk!