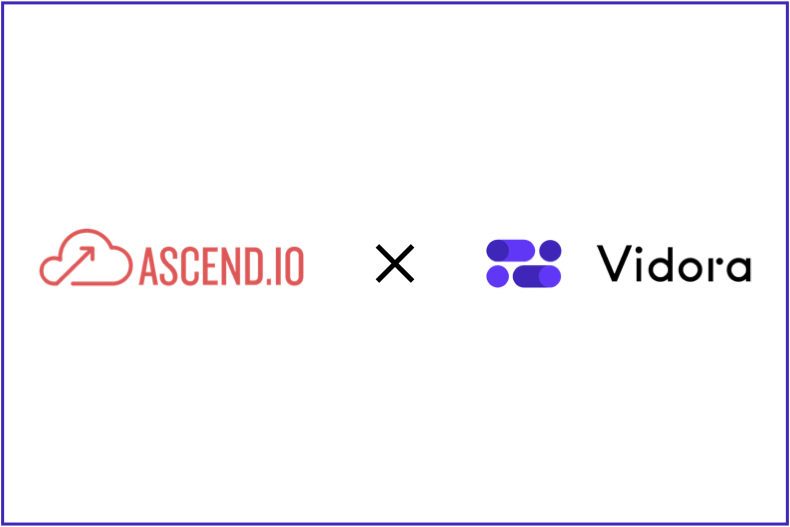
The application of Machine learning to the automation and optimization of various business processes is one of the most impactful ways for businesses to realize ROI from their data. Given the wealth of data captured by marketing and product teams, and the fact that data fuels all Machine Learning technology, these teams are in a prime position to leverage machine learning to drive higher user engagement through 1:1 personalization.
However, typically there are significant organizational challenges when a business operationalizes machine learning. These challenges include:
By combining technology like Ascend’s declarative data pipelines platform with Vidora’s no-code Machine Learning platform, a business can address the challenges described above with even modest teams of data professionals.
Ascend – Reliable Declarative Data Pipelines
As a first step, the Ascend Unified Data Engineering platform enables teams with minimal data engineering skills to build reliable data pipelines that pull data from a variety of sources and send that data to a variety of destinations. Between the sources and destinations, the powerful Ascend Data Aware™ transformation engine with flex-code capabilities transforms raw data into formats and continuous data flows amenable for machine learning. The combination of reliable and consistent data pipelines, with the ability to easily transform and combine many inputs, is an ideal precursor for fully operationalized and highly automated machine learning pipelines.
Screenshots from Ascend, showing flexible data unification and data pipelines
Vidora – No-Code Machine Learning for Consumer Data
As a second step, Vidora provides Cortex, a No-Code Machine Learning Platform that focuses on consumer data and is easily integrated into typical marketing and product workflows. Common use cases powered by Cortex include churn predictions, personalized recommendations, prescriptive modeling like Uplift Modeling, dynamic decisioning, and look-alike models.
Screenshots from Vidora’s No-Code Machine Learning Platform Cortex, making it easy to operationalize machine learning in marketing and product workflows.
Example – Combing Ascend and Vidora to Re-Engage Lapsed Users
Combining the power of a reliable data pipeline with a no-code machine learning platform enables a business to realize value from their data quickly and easily. Let’s consider a typical business use case – re-engaging a lapsed user. Re-engaging high-value lapsed users is a powerful way to extend the LTV (lifetime value) of customers, usually at costs that are lower than new customer acquisition . Many businesses seek to provide proactive discounts to the right lapsed users in order to re-engage them with their service, but need to do so in a highly targeted manner to avoid squandering their incentive budgets.
Typically the use case above would involve building two separate machine learning pipelines –
- Predict which lapsed users are high-value users (Vidora Future Events Prediction)
- Prescribe which user will be most enticed with a discount offer (Uplift Modeling)
Both machine learning pipelines require continuous and up-to-date data in order to ensure the right users are engaged.
Building Continuous Data Pipelines with Ascend
Ascend provides the reliable data pipelines across all the data sources needed to inform the machine learning algorithms, including –
Customer Data Platforms
- Data Warehouses containing Transactional Data
- Behavioral Analytics Platforms like Adobe Omniture
- Contextual data like weather, location, hardware platforms, and more
- Product information, ERP, logistics systems and more
In addition Ascend easily combines data flows from the various sources, transforms the data according to business logic coded by the data team, and delivers the results into a format amenable to machine learning. In the case of re-engaging lapsed users the format would be :
These events are then continuously streamed into Vidora Cortex through the direct integration between Ascend and Vidora to provide the most up-to-date data for the machine learning pipelines.
Building a Predictive Machine Learning Pipeline with Vidora
Cortex allows companies to easily transform customer data into machine learning pipelines, which includes
- Predicting the future behavior of users
- Finding Look Alikes to find more high value users
- Recommending content personalized to each user
- And more
Predicting which lapsed users are most likely to re-engage can be done with a Future Events Pipeline. This pipeline type allows you to choose any event a user can take and predict how likely each user is to take that specific action within a certain time frame.
Example of a Future Events Machine Learning Pipeline in Cortex.
This generates a machine learning pipeline for all users who have churned from a subscription service, and gives each user a probability score indicating their likelihood of re-subscribing to the service within 7 days. This information can then be used to create personalized outreach campaigns to try and win back the business of these users.
It’s Never Been Easier to Operationalize Machine Learning
Combining powerful data science platforms like Ascend and Vidora is making it easier for businesses to quickly deploy machine learning technology. Leveraging these platforms ensures that not only can machine learning models be built by smaller and more diverse teams, but that these models can be reliably deployed within a business to drive value.
To learn more about the partnership between Ascend and Vidora or about the individual platforms please email partners@ascend.io or partners@vidora.com.