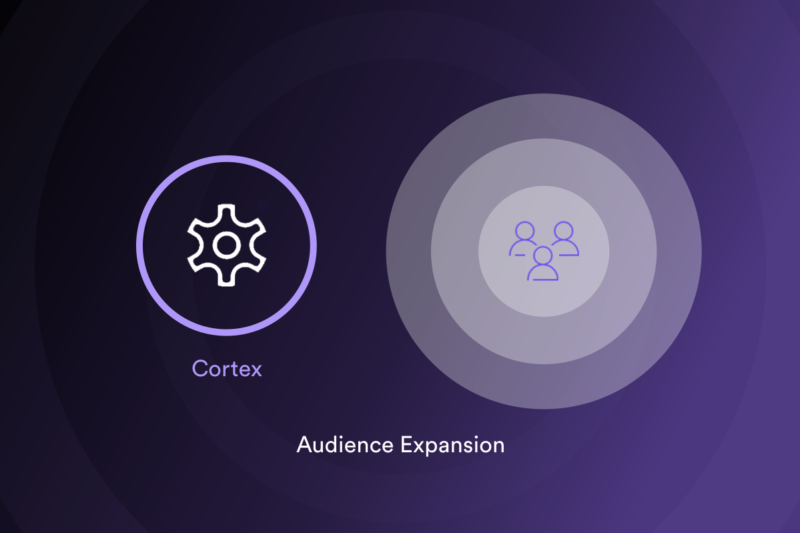
Data is changing the game for marketers. In today’s age of ubiquitous digital content, customers are bombarded with generic marketing messages and advertisements everywhere they go. How can your business stand out from the crowd? What about when tackling audience expansion?
Personalized marketing lets you reach customers in highly targeted ways that boost your chances of attracting and retaining users. According to Constellation Research, lack of content relevancy generates 83% lower response rates in the average marketing campaign. This is no surprise when you consider that in a Marketo survey, 79% of consumers indicated that they are only likely to engage with an offer or promotion if it has been personalized.
In this post we’ll show you how anyone can use survey data to expand audience segments. This facilitates personalization, which takes the guesswork out of your marketing efforts. And with Cortex, this whole process takes less than a minute.
Enabling Personalization with Surveys
Personalized marketing starts with knowing who your customers are and what they like. The most direct way to gather this kind of data is simply to ask. Surveys offer an affordable, reliable, and transparent method of obtaining all kinds of user information, from demographics to preferences and more.
But there’s a catch – even the best surveys typically garner low response rates. Traditionally this has limited the efficacy of survey data. As a result, marketers have to make sweeping assumptions or resort to blunt customer profiles for the majority of their users.
Machine Learning is changing all that, breathing new life into the value of simple yes/no survey data. Audience expansion techniques are enabling marketers to take information collected from a few customers, and leverage it into broad insights about their entire user base. It’s as if you got every user to fill out a detailed survey, so you know exactly how to reach them and with what content.
Machine Learning and Audience Expansion
Generally speaking, Machine Learning algorithms learn from examples to find patterns in data. But different ML algorithms are optimized to solve different problems, and the ones you should use depend on what form your survey data takes.
Say you run a survey which splits respondents into two groups: the “yeses” and the “nos”. Now, you want to learn which users that didn’t take the survey belong in which group. In ML terms, this problem translates to the standard Classification framework.
An ML Classification problem involves assigning data points to one of two classes (positive and negative). To do this effectively, Classification algorithms must learn from both types of examples. For instance, a Classification model which predicts whether a customer is likely to be a homeowner must learn from examples of other customers who are either known to be homeowners (+) or known not to be homeowners (-).
Sometimes, though, your survey responses will provide you with only one group of users. All is not lost – Look Alike modeling (more formally known as one-class classification) is a Machine Learning technique in which your model learns to find data points that are similar to your positive set, even without accessing a corresponding negative set. It’s a tough problem – you’ve got half as much information – but many ML algorithms are sophisticated enough to do the job.
Building these Models in Cortex
Cortex’s simple and intuitive interface allows anyone to build complex Classification and Look Alike models in minutes, even if you have no programming or data science background. Just follow the five easy steps below.
1. Select a model type – create a new pipeline to either Find Look Alikes or Classify Objects, depending on what data you have at your disposal.
2. Upload your sets – Drag and drop a list of users in your positive set and another in your negative set, based on the survey results you collected. If you’re building a Look Alike model, you’ll only have to upload positive labels.
3. Define your dates – User traits can change over time. Someone who wasn’t a homeowner when you ran your survey 6 months ago may have bought a home recently. Specify the range of dates to run your survey. By doing so, you’re letting Cortex know which data to train over.
4. Specify settings – Give your model a descriptive name. Next, apply some tags so that you can easily access it later on.
5. Review and train – Review your model and let Cortex go to work!
Cortex automates every step of the ML pipeline. Now, you can focus on applying it to your business’s most pressing problems. Starting with raw unstructured data, Cortex finds the best path through dozens of Data Preprocessing, Feature Cleaning, Feature Engineering, and Model Selection techniques to optimize the performance of your pipeline. What’s more, our system gets better with every new problem it sees. This all happens behind the scenes, freeing you up to focus your expertise on business challenges rather than data details.
Conclusion
Marketers are constantly looking for ways to identify new users and learn more about existing ones. Cortex makes it easy to build sophisticated Look Alike models. Now you can maximize the reach and efficacy of your audience expansion efforts. Learn more about creating a Look Alike model for your business by emailing support@vidora.com today!